What is the Role of Machine Learning (ML) in Healthcare Software Development?
What is the Role of Machine Learning (ML) in Healthcare Software Development
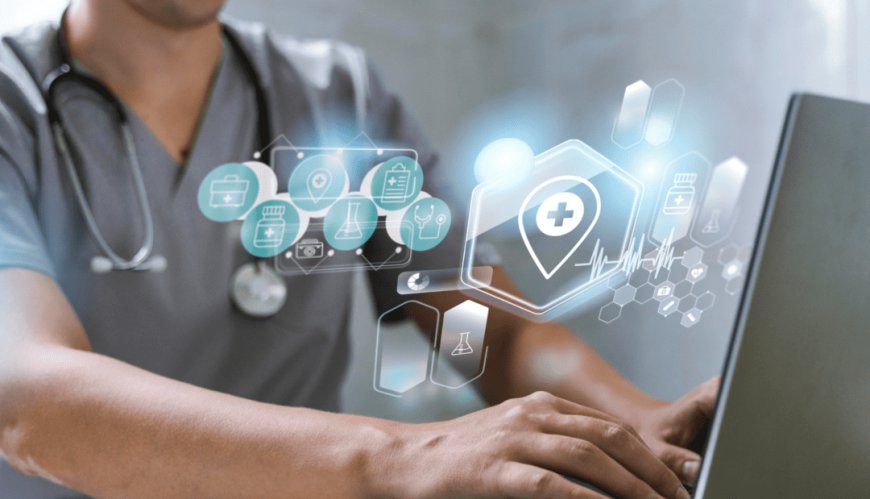
Machine Learning (ML) is revolutionizing the healthcare industry by introducing data-driven solutions that improve the quality of care, accelerate medical research, and enhance operational efficiency. As a subset of Artificial Intelligence (AI), ML focuses on enabling software systems to learn from data and make predictions or decisions without explicit programming. In healthcare software development services, ML is playing a critical role in transforming how healthcare providers manage patient care, conduct research, and handle administrative tasks.
With the ability to process vast amounts of data, detect patterns, and make accurate predictions, ML is making healthcare more efficient and personalized. From medical imaging to drug discovery, ML algorithms are integrated into healthcare software to automate processes, reduce human errors, and improve overall patient outcomes. In this article, we explore the various roles of ML in healthcare software development and how it is shaping the future of healthcare.
1. Medical Imaging and Diagnostics
One of the most prominent applications of ML in healthcare is its ability to enhance medical imaging and diagnostics. Traditionally, diagnosing medical conditions such as cancer, heart disease, or neurological disorders requires expert interpretation of imaging data like X-rays, MRIs, and CT scans. However, ML-powered healthcare software can analyze these images more quickly and accurately than human radiologists in many cases.
-
Image Analysis: ML algorithms can identify abnormalities in medical images with high precision, detecting conditions like tumors, fractures, or internal bleeding at an early stage. For example, deep learning algorithms, a subset of ML, can be trained to recognize specific patterns in thousands of images, improving diagnostic accuracy and helping healthcare professionals make better-informed decisions.
-
Automated Detection: ML models integrated into healthcare software development services can automatically flag potential areas of concern, allowing radiologists to focus on the most critical cases. This speeds up the diagnostic process and reduces the likelihood of missed diagnoses.
By leveraging ML for medical imaging, healthcare providers can reduce diagnostic errors, improve patient outcomes, and optimize the use of medical resources.
2. Predictive Analytics for Early Disease Detection
Predictive analytics is one of the most powerful uses of ML in healthcare software development. ML models are designed to analyze large datasets, detect patterns, and predict future outcomes. In healthcare, predictive analytics helps identify patients at risk for developing specific conditions, enabling early intervention and preventive care.
-
Chronic Disease Prediction: ML algorithms can predict the likelihood of patients developing chronic conditions such as diabetes, heart disease, or hypertension based on their medical history, lifestyle factors, and genetic data. By analyzing patient data, ML can identify early warning signs and suggest interventions before the disease progresses.
-
Population Health Management: ML models can also be used for population health management by identifying trends and risks within a large group of patients. Healthcare providers can use these insights to develop targeted prevention programs, allocate resources effectively, and reduce the overall healthcare burden.
In healthcare software development services, predictive analytics powered by ML is driving the shift toward proactive, data-driven healthcare, helping providers focus on prevention rather than treatment.
3. Personalized Medicine and Treatment Optimization
ML plays a crucial role in personalized medicine, which aims to tailor medical treatments to individual patients based on their unique characteristics. This approach is in stark contrast to the one-size-fits-all treatment plans traditionally used in healthcare. ML-powered healthcare software can analyze a patient’s medical history, genetic makeup, and response to previous treatments to recommend personalized therapies.
-
Genomics and Personalized Care: ML algorithms are used to process genomic data to predict how patients will respond to specific treatments or medications. This enables healthcare providers to develop individualized treatment plans that are more likely to succeed, reducing the need for trial-and-error approaches.
-
Treatment Outcome Prediction: ML models can predict how patients will respond to certain treatments based on their past medical records and demographic data. This allows doctors to adjust treatment plans in real time, improving patient outcomes and reducing the chances of adverse reactions.
By integrating ML into healthcare software development services, providers can offer personalized, evidence-based care that improves patient satisfaction and outcomes.
4. Drug Discovery and Development
The process of discovering new drugs is time-consuming and expensive, often taking years and costing billions of dollars. ML is significantly accelerating this process by analyzing complex biological data, identifying potential drug candidates, and predicting how these drugs will interact with the human body.
-
Identifying Drug Candidates: ML algorithms can sift through large datasets of chemical compounds, biological data, and clinical trial results to identify promising drug candidates. These algorithms help researchers prioritize compounds that are more likely to be effective, reducing the time and cost associated with drug discovery.
-
Predicting Drug Efficacy and Safety: ML models can simulate how new drugs will interact with biological systems, predicting potential side effects and efficacy before clinical trials. This allows pharmaceutical companies to optimize their research and development processes, improving the chances of bringing successful drugs to market.
Incorporating ML into healthcare software development services not only accelerates the pace of drug discovery but also reduces the risks and costs associated with developing new treatments.
5. Natural Language Processing (NLP) for Medical Records and Clinical Data
Natural Language Processing (NLP), a branch of ML, is particularly useful for processing unstructured data, such as clinical notes, medical records, and research papers. Healthcare providers often generate large amounts of unstructured data that can be difficult to analyze using traditional methods. NLP-enabled healthcare software can automatically extract valuable insights from this data, improving decision-making and patient care.
-
Clinical Data Extraction: NLP tools can analyze clinical notes, patient histories, and lab reports to extract relevant information, such as diagnoses, medications, and treatment plans. This structured data can then be used to improve patient care, conduct research, and optimize healthcare workflows.
-
Research and Literature Review: NLP algorithms can also analyze vast amounts of medical literature to identify trends, correlations, and potential new treatments. This helps researchers stay up-to-date with the latest findings and make data-driven decisions in their studies.
With NLP integrated into healthcare software development services, providers can harness the power of unstructured data to improve patient care and accelerate medical research.
6. Enhancing Clinical Decision Support Systems (CDSS)
Clinical Decision Support Systems (CDSS) are designed to assist healthcare providers in making more informed clinical decisions by analyzing patient data and offering recommendations. ML models integrated into CDSS can process vast amounts of information in real time, providing healthcare professionals with valuable insights that improve decision-making and patient outcomes.
-
Real-Time Decision Support: ML-powered CDSS can analyze patient data in real time to suggest potential diagnoses, treatment plans, and risk factors. These systems can alert physicians to potential drug interactions, recommend alternative therapies, and even suggest additional tests based on the patient's condition.
-
Reducing Diagnostic Errors: By offering data-driven recommendations, ML-enhanced CDSS reduces the risk of diagnostic errors and ensures that healthcare providers have access to the most accurate and up-to-date information when making decisions.
Incorporating ML into healthcare software development services ensures that healthcare professionals are equipped with the tools they need to make better decisions, ultimately improving patient outcomes and reducing medical errors.
7. Optimizing Hospital Operations and Resource Management
In addition to clinical applications, ML is also being used to improve hospital operations and resource management. Healthcare institutions face significant challenges in managing resources efficiently, from staffing and scheduling to inventory management and patient flow. ML-powered healthcare software can analyze operational data to optimize workflows and improve efficiency.
-
Patient Flow Optimization: ML algorithms can predict patient admissions, discharges, and transfers, helping hospitals manage patient flow more effectively. By forecasting patient demand, healthcare facilities can optimize staffing levels, reduce wait times, and ensure that resources are allocated appropriately.
-
Inventory and Supply Chain Management: ML models can analyze supply chain data to predict demand for medical supplies, ensuring that hospitals have the necessary equipment on hand without overstocking. This helps healthcare providers reduce costs and improve efficiency.
By integrating ML into healthcare software development services, hospitals and healthcare organizations can optimize their operations, reduce costs, and provide better patient care.
Conclusion
Machine Learning is playing an increasingly important role in healthcare software development services, offering data-driven solutions that improve diagnostics, personalize treatment, accelerate drug discovery, and optimize hospital operations. As healthcare becomes more data-driven, ML's ability to analyze large datasets, detect patterns, and make accurate predictions is transforming the way healthcare providers deliver care.
From enhancing clinical decision-making to improving hospital efficiency, ML is enabling healthcare providers to offer more personalized, proactive, and efficient care. As the healthcare industry continues to evolve, the integration of ML into healthcare software development will only deepen, unlocking new possibilities for improving patient outcomes and reducing the overall cost of healthcare delivery.