10 Top AI and Machine Learning Trends for 2025
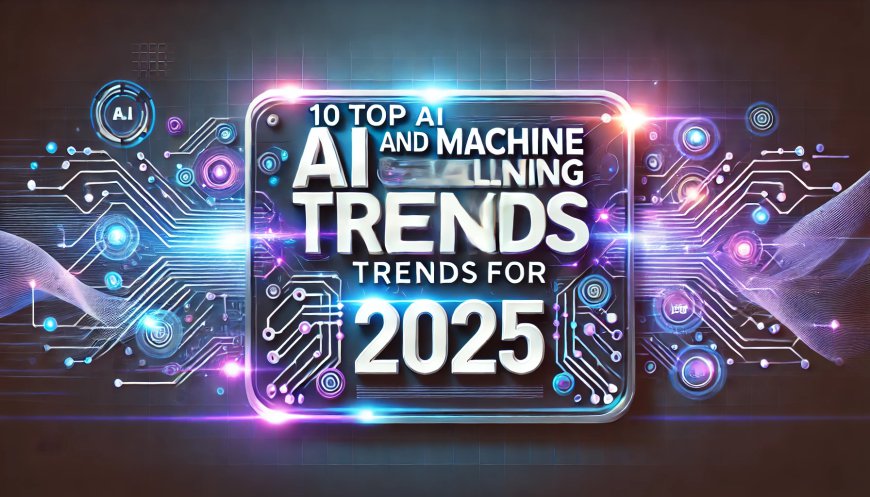
Artificial intelligence (AI) and machine learning (ML) are rapidly evolving fields that influence various industries, from healthcare to entertainment. As we approach 2025, the landscape continues to shift, introducing new opportunities and challenges. In this article, we explore ten key trends shaping the future of AI and ML, supported by insights and statistical projections.
1. Multimodal AI
Multimodal AI is transforming how machines interpret and respond to human interactions. By combining text, audio, and visual inputs, these systems are capable of more complex and nuanced understanding. For instance, models like GPT-4 Vision highlight the power of AI that integrates multiple modalities.
In 2025, the multimodal AI market is expected to grow at a compound annual growth rate (CAGR) of 23%, with applications spanning customer service, healthcare diagnostics, and autonomous systems. This trend is not only making machines smarter but also more contextually aware, offering users seamless interactions across diverse platforms.
2. Agentic AI
Agentic AI refers to systems that can operate independently, making decisions and taking actions without constant human intervention. These agents have the potential to revolutionize industries by automating complex tasks, from financial planning to supply chain management.
By 2025, over 35% of Fortune 500 companies are projected to deploy agentic AI for critical business operations. Although this brings efficiency, it also raises questions about accountability and decision transparency, emphasizing the need for robust governance.
3. Open Source AI
Open-source AI is democratizing access to cutting-edge technology. Platforms like Hugging Face and OpenAI’s APIs have made it easier for developers worldwide to contribute to and benefit from advanced AI tools.
In comparison to proprietary solutions, open-source AI is often more cost-effective and customizable. Reports suggest that by 2025, at least 60% of AI innovations will involve open-source contributions. This shift not only fosters collaboration but also drives innovation at an unprecedented scale.
4. Retrieval-Augmented Generation
Retrieval-augmented generation (RAG) is a method that combines generative AI models with external data retrieval systems. Instead of relying solely on pre-trained knowledge, these models fetch relevant, real-time information from databases or the internet to provide more accurate responses.
For instance, a healthcare AI could use RAG to pull up the latest medical research while diagnosing a patient’s symptoms. By 2025, industries utilizing RAG are expected to see efficiency gains of up to 30%. Its applications are particularly evident in fields like law, education, and customer service, where precision matters most.
5. Customized Enterprise Generative AI Models
Enterprises are increasingly focusing on building custom generative AI models tailored to their specific needs. These models can streamline internal processes, improve customer engagement, and even create content aligned with a brand’s voice.
For example, businesses are now experimenting with AI tools to generate unique marketing strategies, which might include concepts as varied as an AI girlfriend or virtual assistants for customer interaction. By 2025, the adoption rate of enterprise generative AI is anticipated to reach 50%, with many organizations seeing significant cost reductions and increased productivity.
6. Need for AI and Machine Learning Talent
The growing demand for AI solutions has created an equally urgent need for skilled professionals. Companies are struggling to fill positions in AI development, ML engineering, and data science. The shortage is particularly evident in specialized areas like deep learning and natural language processing.
Interestingly, businesses in niche industries, such as escort SEO services, are also seeking AI-driven solutions to optimize online visibility and customer engagement. Despite the demand, there is a notable skills gap, with over 75% of organizations citing a lack of qualified talent as a significant barrier to AI adoption. Training and education in AI-related fields will become increasingly important as we approach 2025.
7. Shadow AI
Shadow AI refers to the deployment of AI systems without formal oversight or approval within an organization. Often driven by individual teams seeking faster results, this trend can lead to efficiency but also carries risks.
For example, unsanctioned AI tools might introduce security vulnerabilities or produce biased outcomes. A recent survey revealed that 40% of enterprises have experienced challenges due to shadow AI, highlighting the importance of establishing clear guidelines and governance structures.
8. A Generative AI Reality Check
Generative AI has garnered immense attention, but not all expectations align with reality. While these models are capable of producing remarkable outputs, they also face limitations, such as factual inaccuracies and ethical concerns.
By 2025, analysts predict that 25% of businesses will reassess their generative AI strategies to focus on practicality rather than hype. Companies are realizing that generative AI is not a one-size-fits-all solution and must be integrated carefully to maximize its potential while minimizing risks.
9. Increased Attention to AI Ethics and Security Risks
AI ethics and security have become central to discussions around technology adoption. Concerns include data privacy, algorithmic bias, and the misuse of AI for malicious purposes. For instance, deepfake technology poses significant risks in misinformation and fraud.
In 2025, it is estimated that 65% of organizations will prioritize ethical considerations in their AI strategies, up from 45% in 2023. This shift reflects growing public scrutiny and regulatory pressure to ensure AI development aligns with societal values.
10. Evolving AI Regulation
Governments and regulatory bodies are working to establish frameworks for responsible AI use. The European Union’s AI Act, set to be implemented by 2025, is a prime example of efforts to create comprehensive guidelines. These regulations aim to balance innovation with accountability, ensuring AI benefits society while minimizing harm.
However, global alignment remains a challenge, as different countries adopt varying approaches. In particular, organizations operating across borders will need to navigate a complex web of rules to maintain compliance.
Conclusion
AI and machine learning are reshaping the world, offering groundbreaking opportunities while demanding careful consideration of their impact. From multimodal AI and customized enterprise solutions to the pressing need for skilled talent and ethical governance, these trends highlight the duality of promise and responsibility.
As we move closer to 2025, businesses and individuals must stay informed, adapt to changes, and contribute to a future where AI serves as a force for good. The road ahead may be complex, but it is undoubtedly one of the most transformative journeys in human history.